Data Science: Bridging the Gap Between Data Analysis and Decision Making
- Data Synergy
- Apr 30, 2024
- 3 min read
In today's data-driven world, businesses are inundated with vast amounts of data. While this data holds immense potential for insights and strategic decision-making, harnessing its power effectively is often easier said than done. This is where data science steps in, acting as a bridge between raw data and actionable insights, thereby facilitating informed decision-making processes. Let's explore how data science serves as this vital connector, bridging the gap between data analysis and decision-making.
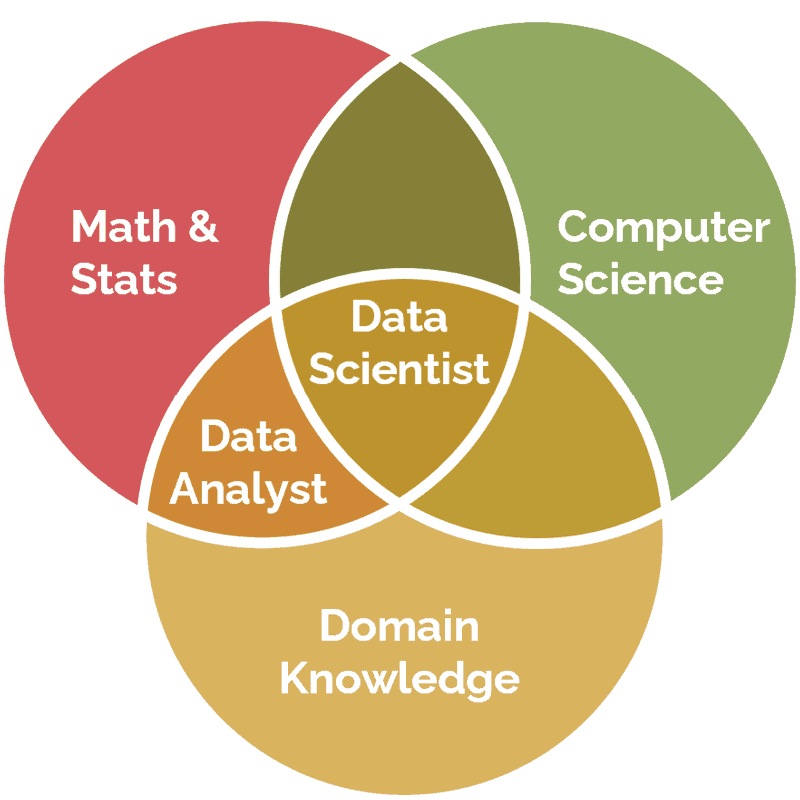
Understanding Data Science
At its core, data science is an interdisciplinary field that combines expertise in statistics, mathematics, computer science, and domain-specific knowledge to extract insights and knowledge from data. Data scientists leverage various techniques, algorithms, and tools to analyze data, identify patterns, and generate actionable insights.

The Data AnalysisProcess
Data analysis is a fundamental component of data science, involving the systematic examination of data to uncover meaningful insights. This process typically includes data collection, data cleaning, exploratory data analysis (EDA), statistical analysis, and machine learning modeling.
Bridging the Gap
Data science serves as a bridge between data analysis and decision-making processes in several key ways:
1. Data Preparation: Data scientists are adept at preparing and transforming raw data into a format suitable for analysis. This involves cleaning, preprocessing, and transforming the data to ensure its quality and relevance.
2. Feature Engineering: Data scientists extract and engineer relevant features from the data to enhance the performance of machine learning models. Feature engineering involves selecting, transforming, and creating new features that capture meaningful information from the data.
3. Model Development: Data scientists leverage machine learning algorithms to build predictive models that can make accurate predictions or classifications based on the data. These models learn from historical data to identify patterns and relationships that inform future decisions.
4. Evaluation and Validation: Data science involves rigorously evaluating and validating the performance of predictive models to ensure their accuracy and reliability. This involves testing the models on unseen data and using metrics such as accuracy, precision, recall, and F1-score to assess their performance.
5. Insights Generation: Ultimately, data science generates actionable insights from the results of data analysis and predictive modeling. These insights provide decision-makers with valuable information that can guide strategic planning, resource allocation, risk management, and operational improvements.
Benefits of Data Science
The application of data science in bridging the gap between data analysis and decision-making brings several benefits:
1. Informed Decision-Making: By leveraging data science, organizations can make informed, data-driven decisions based on empirical evidence rather than intuition or guesswork.
2. Efficiency: Data science automates and streamlines data analysis processes, enabling organizations to analyze large volumes of data quickly and efficiently.
3. Competitive Advantage: Organizations that embrace data science gain a competitive edge by leveraging data-driven insights to innovate, optimize processes, and seize new opportunities.
4. Risk Mitigation: Data science helps organizations identify and mitigate risks by uncovering hidden patterns and trends that may impact business operations or performance.
Challenges and Considerations
While data science offers tremendous potential, it also presents challenges that organizations must address:
1. Data Quality: Ensuring the quality, accuracy, and reliability of data is paramount for effective data analysis and decision-making.
2. Data Privacy and Security: Organizations must safeguard sensitive data and comply with data privacy regulations to protect individuals' privacy and prevent unauthorized access or misuse of data.
3. Interpretability: Complex machine learning models may lack interpretability, making it challenging to understand and trust their predictions.
4. Ethical Considerations: Data science raises ethical considerations related to fairness, bias, and accountability.
In conclusion, Data science plays a pivotal role in bridging the gap between data analysis and decision-making processes. By leveraging data science techniques and methodologies, organizations can extract valuable insights from data, inform strategic decision-making, and drive innovation and growth. However, to unlock the full potential of data science, organizations must address challenges related to data quality, privacy, interpretability, and ethics, ensuring that data-driven decisions are ethical, transparent, and accountable.
In essence, Data Science empowers organizations to harness the power of data to make informed decisions, solve complex problems, and drive positive outcomes in an increasingly data-centric world.
Comments