Responsible AI and Data-Centric AI: Leading the Way in Ethical and Efficient AI Practices
- Data Synergy
- Nov 12, 2024
- 2 min read
Updated: Apr 18
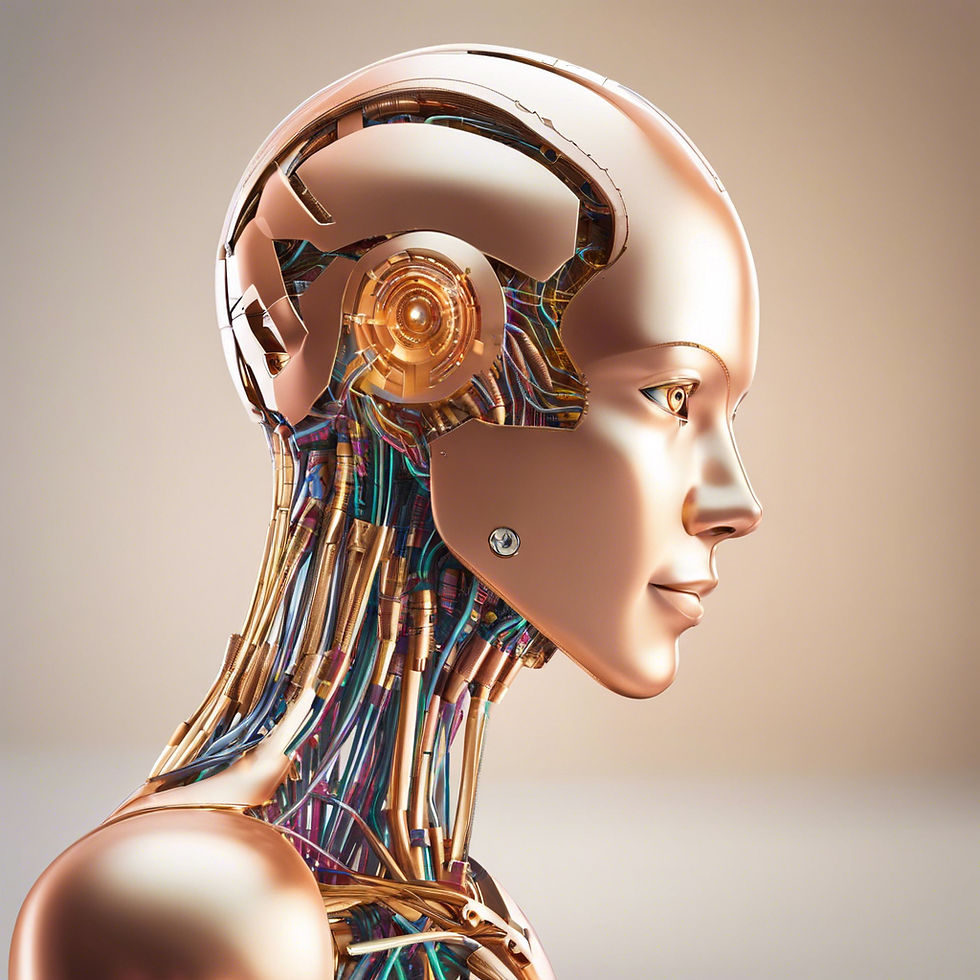
As artificial intelligence (AI) becomes more prevalent across various industries, two key trends—Responsible AI and Data-Centric AI—are emerging as critical to the future of AI development and application. These approaches focus on ethical AI usage and improved data management, significantly impacting how AI systems are created and implemented.
Responsible AI: Promoting Ethical AI Utilization
Responsible AI is centered around the ethical deployment of AI systems. It involves several core principles designed to ensure that AI technologies are developed and used responsibly:
1. Ethical Standards: Responsible AI prioritizes transparency, fairness, and accountability, ensuring that AI decisions are made ethically and free from bias. This builds trust among users and stakeholders.
2. Risk Mitigation: Organizations are advised to adopt a risk-proportional approach to AI implementation, which involves evaluating potential risks and implementing strategies to manage them. This helps ensure AI models comply with legal and regulatory standards, avoiding financial loss, legal issues, and reputational harm.
3. Transparency and Explainability: Making AI systems transparent and explainable is crucial for trust. Users should be able to understand AI decision-making processes, which helps verify fairness and accuracy.
4. Societal Considerations: Responsible AI also addresses the broader societal impact of AI technologies. This includes assessing how AI affects employment, privacy, and human rights, and striving to maximize positive outcomes while minimizing negative effects.
Data-Centric AI: Emphasizing Data Quality and Management
Data-Centric AI shifts the focus from models and algorithms to the importance of high-quality data in AI development. This approach aims to enhance AI system performance through improved data management practices:
1. High-Quality Data: Data-centric AI underscores the necessity of accurate, complete, and relevant data for training AI models. High-quality data is essential for developing robust and reliable AI systems.
2. Synthetic Data: Synthetic data is becoming more popular as a solution to data scarcity and privacy concerns. By creating realistic data sets that replicate real-world data, synthetic data enables effective AI training without compromising privacy.
3. Advanced Data Management: Innovations in data management, such as AI-specific tools and automated data labeling technologies, are integral to data-centric AI. These technologies streamline data processing and improve the efficiency of AI model training.
4. Data Integration and Accessibility: Data-centric AI also involves making data more accessible and easier to integrate across different systems and platforms. This ensures AI models can utilize diverse data sources, enhancing their accuracy and applicability.
In conclusion, both Responsible AI and Data-Centric AI are vital for the future of AI. Responsible AI ensures ethical and transparent use of AI technologies, fostering trust and mitigating risks. Data-Centric AI emphasizes the critical role of high-quality data in building effective AI systems, leading to more accurate and reliable applications.
Adopting these approaches allows organizations to leverage AI's full potential while addressing ethical concerns and improving data management practices. This dual focus not only enhances AI system performance but also ensures that AI technologies are developed and deployed for the greater good.
Comments